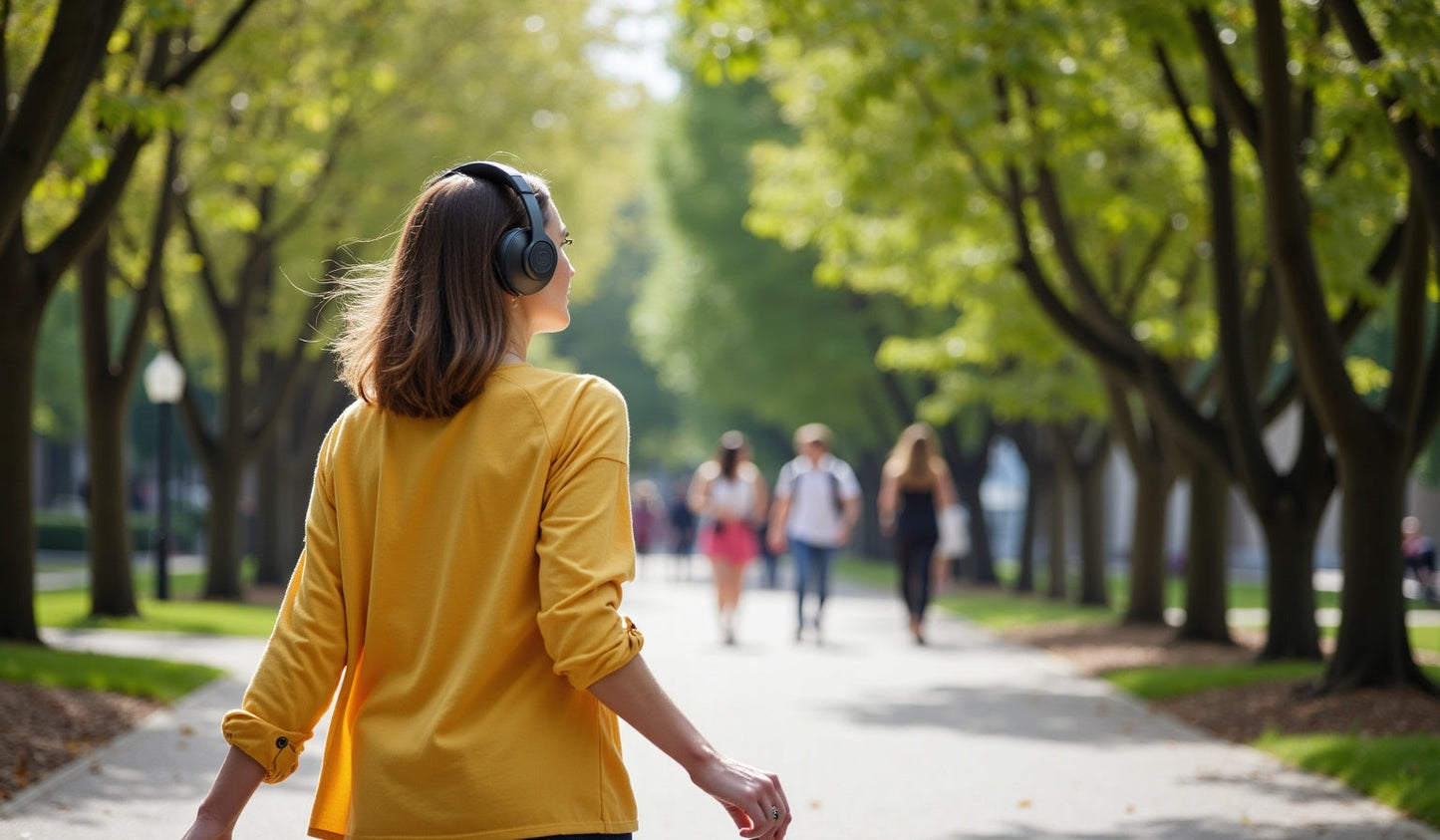
What if your headphones could sense rising stress before you felt overwhelmed—and automatically adjust the soundscape to help you feel calm and centered again? Or if your daily tools could detect patterns of distraction and help you refocus in real time?
This is no longer science fiction. Thanks to breakthroughs in electroencephalography (EEG), we’re entering a new era of emotional intelligence—where wearable technology can not only track how you feel, but help guide your mental state in the moment.
Just a few decades ago, EEG was confined to hospitals and research labs. Originally developed in the 1920s, EEG was used to study epilepsy, sleep disorders, and basic neural function under tightly controlled conditions. The machines were bulky, expensive, and required conductive gels and trained technicians to operate. Fast forward to today, and the same core technology is now embedded in sleek, wireless devices—bringing real-time brain tracking out of the lab and into the hands (and heads) of everyday users.
In this post, we’ll break down how EEG data reflects emotional states, what the latest research reveals, and how this technology is transforming personal wellbeing through practical, real-time tools.
What Is EEG—and Why It Works
EEG measures the brain’s electrical activity, using sensors to detect patterns called brainwaves. Unlike facial expression tracking or voice analysis, EEG goes straight to the source—your neural activity.
Compared to other methods of tracking emotional and mental states, EEG offers a unique combination of speed, cost-effectiveness, and portability. Functional MRI (fMRI), for instance, provides detailed spatial maps of brain activity but is expensive, slow, and immobile—making it unsuitable for real-time feedback or everyday use. Heart rate variability (HRV), another popular measure, offers indirect insights into emotional state via the autonomic nervous system, but lacks the moment-to-moment neural resolution of EEG.
This makes EEG especially well-suited for tracking fast-changing cognitive and emotional states in real time. Its direct measurement of brain signals allows for the development of tools that can respond within seconds—enabling truly interactive mental fitness applications.
Because each mental state corresponds to a different frequency band, EEG can detect subtle shifts that might otherwise go unnoticed. For instance:
-
Delta (0.5–4 Hz): Deep sleep and unconscious processes
-
Theta (4–8 Hz): Drowsiness, meditation, emotional integration
-
Alpha (8–12 Hz): Calm, quiet focus
-
Beta (12–30 Hz): Alertness, active thinking, stress
-
Gamma (30+ Hz): Complex thought, problem-solving, insight
As machine learning continues to evolve, these patterns are being mapped with increasing precision—allowing tools to translate raw EEG data into actionable emotional insights. The result? A growing class of wearables and software platforms that don’t just monitor how you’re doing—they respond in real time to help shift you toward a healthier mental state.
What Emotional States Look Like in Brain Data
While EEG has been around for over a century, its everyday applications are just beginning to take shape. Today’s researchers are refining models that can identify emotional states—like stress, relaxation, focus, or fatigue—based on shifts in your brain’s electrical signals.
To appreciate how these findings are shaping the world beyond the lab, it helps to look at where they’re already showing up. In therapy, clinicians use these insights to deliver neurofeedback that reinforces healthier mental patterns. In wellness and performance coaching, they support training programs focused on emotional regulation and resilience. Even in entertainment—think gaming, film, or advertising—developers are applying EEG-informed design to better understand audience reactions and optimize emotional engagement.
Recent EEG research has uncovered key brainwave patterns linked to various emotional and cognitive states:
-
Frontal alpha asymmetry has emerged as a possible biomarker for mood regulation and may help detect susceptibility to depression.
-
Elevated theta activity has been associated with both meditative states and emotional disengagement, depending on the broader context of neural activity.
-
Beta and gamma activity often increase during periods of high mental load, alertness, or anxiety—and are seen as useful indicators for arousal and attentional shifts.
While these findings are often discussed in clinical or academic settings, their relevance is growing in tech-driven wellbeing platforms. As machine learning models become better at interpreting these signals in real time, they're being translated into actionable feedback for users—supporting everything from mental health interventions to personalized focus tools.
These brainwave-emotion links aren’t just theoretical—they’re already being used in a range of practical settings. In clinical therapy, EEG is employed as part of neurofeedback protocols to help individuals regulate mood or reduce anxiety. In biofeedback training, real-time EEG data can guide users to achieve desired states like calm or alertness more consistently. Even industries like gaming and advertising are leveraging emotional EEG data to evaluate user engagement and emotional resonance, helping design more immersive experiences.
Much of this progress in emotional EEG research has been enabled by large-scale, publicly available datasets. For example, DEAP (Dataset for Emotion Analysis using Physiological Signals) provides multimodal recordings of participants watching music videos, enabling researchers to link physiological signals—including EEG—to self-reported emotional states. SEED (SJTU Emotion EEG Dataset) goes further by offering repeated recordings from the same individuals across different sessions, helping scientists examine emotional consistency and variability over time. DREAMER offers portable EEG recordings tied to self-assessed arousal and valence, making it especially relevant for real-world, mobile EEG applications.
These datasets are more than academic tools—they are the foundation for training machine learning models that power emotion-aware technologies. By teaching algorithms to recognize brainwave patterns tied to joy, stress, boredom, or focus, these resources are helping pave the way for emotionally responsive systems that adapt in real time to support our cognitive and emotional needs.
Making EEG Useful: The FACED Dataset
To make EEG practical for everyday use, researchers need better tools and more naturalistic data. One important contribution is the FACED dataset—short for Fine-grained Affective Computing with EEG Dataset, a project from Southeast University and the Chinese Academy of Sciences.
FACED recorded EEG signals from over 120 participants as they reacted to emotionally powerful video clips. By focusing on real, spontaneous reactions—not artificial lab tasks—it created a rich foundation for training machine learning models.
Using advanced techniques such as differential entropy (DE) to extract meaningful statistical features from EEG signals, and contrastive learning to align neural patterns across different individuals, researchers were able to improve the accuracy and generalizability of emotion recognition models. These methods help account for the wide variability in how emotions manifest in different brains—a key hurdle in real-world EEG applications. In the FACED dataset, models trained with this approach achieved classification accuracies of up to 79% within individual users and approximately 69% across users, demonstrating both high sensitivity and promising cross-subject performance. This marks a significant advance in building more adaptive, personalized emotion-aware technologies.
Beyond its academic value, FACED is helping shape how commercial EEG products are trained and evaluated. By offering a high-quality, emotionally grounded dataset, it’s giving product developers the raw material needed to refine emotion-sensing algorithms, create more accurate feedback systems, and set benchmarks for reliability and user responsiveness in consumer neurotech.
The Future of Mental Fitness: Real-Time Emotional Feedback
This kind of emotional tracking isn’t just for labs anymore. Thanks to wearable EEG tech and AI, these tools are becoming part of everyday life—giving us more awareness and control over how we feel and perform.
By integrating emotional state recognition into daily routines, EEG-based tools can help us catch early signs of cognitive overload or emotional dysregulation. Imagine receiving a gentle prompt to take a break when your brain starts to show signs of fatigue, or being nudged toward a calming soundscape when rising beta activity suggests heightened stress. These kinds of micro-adjustments can have meaningful long-term impacts on mental clarity, emotional regulation, and overall wellbeing.
For example:
-
Detecting cognitive fatigue during a long work session
-
Spotting rising stress levels in real time
-
Reinforcing relaxation during a meditation or wind-down period
This is emotional fitness in action—developing the ability to understand, regulate, and train your brain to respond in healthier ways.
Looking ahead, the potential becomes even more powerful when EEG is combined with other biosignals. Integrating facial recognition for micro-expressions, vocal tone analysis, or even heart rate variability could create a more holistic picture of emotional state. This kind of multimodal fusion is likely to define the next generation of neuroadaptive technologies—offering not just reactive tools, but deeply personalized support for mental wellbeing across every part of daily life.
enophones and the Rise of Adaptive Audio
One of the most exciting examples of this technology in the real world is enophones—a wearable EEG platform that turns your headphones into a mental fitness device.
Using built-in EEG sensors, enophones continuously monitor your brainwave activity. The eno platform then adapts your audio environment based on your mental state—helping you focus, relax, or reset depending on what your brain needs in the moment.
At the heart of the eno platform is a closed-loop system that actively engages with your brain in real time. It begins by reading your brain’s electrical activity using the EEG sensors embedded in the headphones. This neural data is analyzed to detect your current mental state—whether you’re focused, fatigued, or in need of relaxation. Based on this input, the platform adjusts your soundscape dynamically, selecting audio elements designed to steer you toward a desired state. Then, it re-reads your brain’s activity to evaluate the impact of that adjustment, fine-tuning the experience accordingly. This continuous feedback loop creates an experience that is both personalized and adaptive, supporting deeper focus, emotional regulation, and sustained mental clarity.
Over time, this creates a personalized experience that helps you train your attention, build emotional resilience, and develop healthier patterns of brain activity.
If you’ve ever wished for a soundtrack that gets you—this is it.
Explore more at getenophone.com.
References
To further explore the science and datasets underpinning emotion recognition in EEG research, here are several foundational resources:
-
Koelstra et al. (2012) — DEAP: A Dataset for Emotion Analysis Using Physiological Signals. This benchmark study introduced a multimodal dataset combining EEG, facial expressions, and physiological responses as participants viewed music videos designed to evoke emotional responses. It has since become a key training resource for affective computing models.
-
Zheng & Lu (2015) — Investigating critical frequency bands and channels for EEG-based emotion recognition. This paper identifies which EEG features—such as specific frequency bands or scalp regions—are most predictive for classifying emotional states, offering crucial guidance for hardware and algorithm development.
-
Li et al. (2022) — FACED: A Fine-grained EEG Dataset for Emotion Recognition. This dataset stands out for its focus on spontaneous emotional reactions using real-world video stimuli, and it introduces modern machine learning techniques such as contrastive learning for improved cross-subject generalization.
Together, these references illustrate the interdisciplinary evolution of emotion-aware neurotechnology—from signal processing and experimental psychology to real-time machine learning and consumer product development. They also highlight the growing emphasis on ecological validity: capturing data that reflects genuine human emotion in natural contexts, rather than sterile lab environments. This shift is essential as EEG-driven tools become increasingly integrated into everyday life.